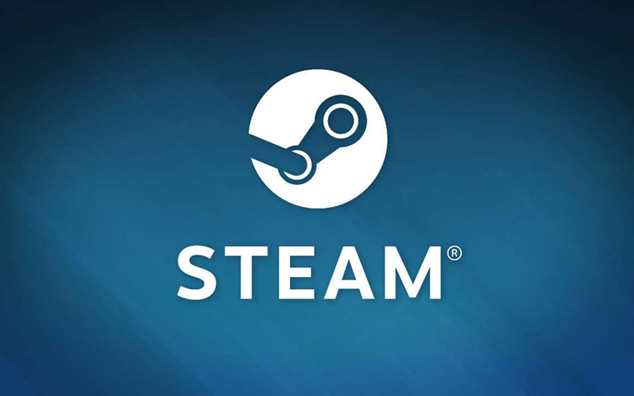
Steam is a well-known online video game service that has triumphantly risen to the top as one of the best, if not the best, video game services on the internet. While most gamers think it is due to the fact that Steam predominantly runs on PC/Computers (the largest group of gaming consumers on the market), I think the reason for its success lies within its recently developed algorithm.
After a recent fiasco of giving users and game developers direct control over the “tags” used by the algorithm, Valve (The company that created Steam) redeveloped its recommendation algorithm to prevent user exploitation while still balancing user preferences and focusing on target audiences. This post is a brief summary of Steam’s transformation.
The Problem – Tags and Reviews
Previously, Steam’s gaming service’s way of recommending games to gamers was through the implementation of tags, the same as Goodreads. These “tags” were generated by users and game developers, and they were intended to depict the concepts of the games. This way, when a user looks for a specific game or genre of games, the recommendation algorithm would list the options that had tags that matched the queries. In addition to this, game developers were able to highlight certain reviews that they thought would depict the game well for new users. Giving this much power to users and game developers was the root cause of the exploitation problem.
Game developers started to insert tags that weren’t even related to the game, typically tags that were trending currently, in order to get it recognized by the algorithm and placed in the “Popular” category. Furthermore, game developers would choose to highlight only 5-star reviews, which lead to the algorithm assuming that the game was more favorable than it really was. As the Senior gaming editor at Ars Technica Kyle Orland put it, developers were “choosing popular tags or leaning on positive reviews” to artificially boost their sales, popularity, and performance (Orland 2). This led to many gamers getting cheated into believing that the game they had paid for and downloaded was what they were promised.
On the other hand, gamers had also started to exploit the algorithm, by unnecessarily devaluing certain games. Games that were doing good were suddenly getting bombarded by these “review trolls” leaving negative remarks or inappropriate tags, that did not represent the game. Similar to the previous exploitation, this led to many game developers suffering, as their games would never gain in popularity. In addition to that, these games would be hidden from the average gamer’s sight.
Valve knew this was a problem, but they faced several difficulties in attempting to combat it. If they implemented a strict or heavily controlled vocabulary of terms, it would decrease how much value the popularity of games had, which would result in Steam performing poorly. If they let this problem continue, it would soon consume all of the recommended sections and only show a select few games that were overly inflated in fake characteristics. In 2019, Valve came up with a bold remodeling of their algorithm, which resolve this issue, while still giving room for popularity.
The Solution – “Interactive Recommender”
The first step to this solution was to give the loyal users a way to bypass the inflated “Recommended” section. This new implementation, visuals below, is called the “Interactive Recommender,” and it uses advanced machine learning algorithms to filter games, tailored to their past game choices and their input cues. It will directly give a searching user control over how the algorithm should search for a game: From popularity level to older or newer and all the potential tags to include or not include.
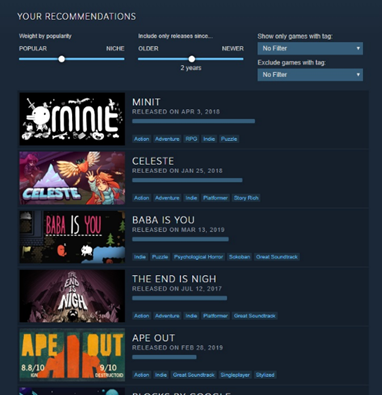
In addition to this, Valve also implemented a checking system for the tags that were added, to filter out the fake tags. This process did take more effort, but it limited the number of fakes while balancing user control.
What this means for the user is that they can now freely explore the entire Steam library and not be stuck looking at the same set of popular/newer games. Valve claimed that “digging into the ‘niche’ end of the range can be a very effective way to find hidden gems” for gamers who don’t want the same old “popular” genres (Orland 2). Previously, if a gamer wanted a specific kind of game, they would have a better chance of finding it only if they knew the exact name of the game.
Playtime vs Reviews
The next installation of the changes to the algorithm was the addition of a “playtime” based recommendation. Since tags and reviews could be easily fooled, it would take more time and effort for Valve to correct every tag, and they decreased its value in the eyes of the algorithm. Instead, a larger chunk of the recommendations came from how many hours players spent on a specific game. The logic behind this was that only if a game was “good” would a player spend hours playing it. This is something unique to games, as they are played continuously rather than viewed, read, or watched once. And this way, only those who played the game’s opinions were represented at large. This made the work of trolls and exploiters much less significant or impactful. Orland remarked that “playtime history is a core part of this neural-network-driven model. The number of hours you put into each game in your library is compared with that of millions of other Steam users so the neural network can make ‘informed suggestions’” (Orland 1).
Though reviews became less valued, they certainly did not disappear. The chart below is an accurate representation of how the algorithm interprets reviews now, which allows for a larger margin of user error or exploitation.
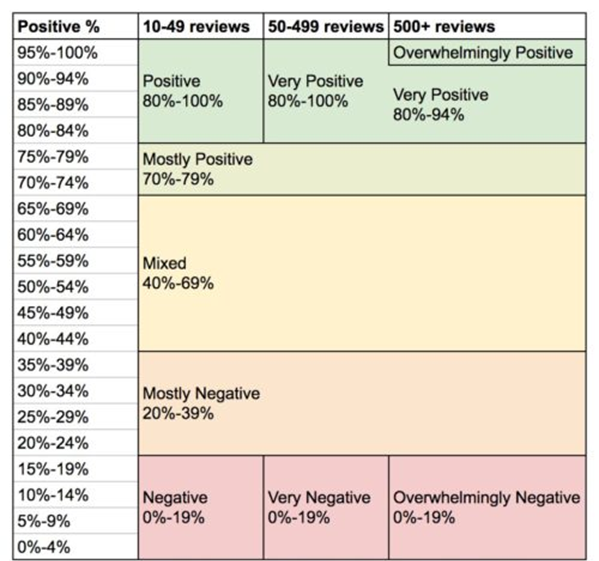
Conclusion
As with any algorithm, this newer implementation is still not perfect. And Valve knows it. They wanted to make the change that would address the immediate concerns, as they work to tinker with the algorithm from the background. These changes are relatively small, which Valve claims is because “Rather than introducing a big change to the way customized recommendations are determined on Steam, we’re introducing this new recommender as an experiment customers can seek out and try. This will help us get better usage data while avoiding any sudden shifts that we know can be frustrating for customers and developers who are accustomed to Steam” (Dingman 6). But one thing is for sure, after these changes, gamers have started to respect and appreciate Steam a lot more than they previously did, which has led to the recent hike in Steam’s performance.
Works Cited
Dingman, Hayden. “Valve’s Intriguing ‘Steam Labs’ Experiments Help You Find New Games in a Sea of Releases.” PCWorld, vol. 37, no. 8, Aug. 2019, pp. 20–23. EBSCOhost, https://search-ebscohost-com.unh-proxy01.newhaven.edu/login.aspx?direct=true&db=f5h&AN=137786761&site=ehost-live&scope=site.
Kamal, Ahmed, et al. “Recommender System: Rating predictions of Steam Games Based on Genre and Topic Modelling,” 2020 IEEE International Conference on Automatic Control and Intelligent Systems (I2CACIS), 2020, pp. 212-218, doi: 10.1109/I2CACIS49202.2020.9140194.
Nesterenko, Oleg. “Steam’s Algorithms Demythologized.” Game World Observer, 23 Sept. 2020, https://gameworldobserver.com/2020/09/23/steam-algorithm.
Orland, Kyle. “Steam Uses Machine Learning for Its New Game Recommendation Engine.” Ars Technica, 11 July 2019, https://arstechnica.com/gaming/2019/07/steam-turns-to-ai-to-help-users-find-gems-amid-thousands-of-games/.