For the last century scientists have been wondering how machines can think. According to Alan Turing humans use available information, and reason to solve problems. So why can’t machines do the same thing? This is a very complicated question which I am not fully qualified to answer, However I do believe that current artificial Intelligence is starting to get close. Modern AI can use information given to it, or in some cases it can find the information on its own. It can then use this information to make predictions and solve problems using reasoning.
DALL-E 2 by OpenAI is a prime example of this. Using several complex algorithms, it can create images from any prompt. You specify what you want the image to contain, and you can even tell the AI what style you want it in. It can make paintings that rival the greatest artists, or images so realistic you can’t tell that it was made by a computer. However just like the old saying “With great power comes great responsibility.” An artificial intelligence this powerful can pose a threat. It is not going to take over the world like in Science Fiction films, but the images created may be used for harm.
DALL-E 2 is extremely complicated, as one would expect given the extremely realistic output. The AI uses a combination of algorithms to produce high-quality images that reflect what the user entered in the prompt. There are two main systems used to generate images. The first is called CLIP. In simple terms, CLIP takes images and text descriptions and tries to match them together. This model has been around since the original DALL-E, which was released in 2021, so it is still relatively new. CLIP is trained on hundreds of thousands of image-text pairs, which are images with a caption.
Something that makes the CLIP model so powerful is its ability to use zero-shot learning. This is when the AI attempts to predict something that wasn’t in the training data. This is extremely important because even if a user types in a prompt that is not in the training data, the AI will still be able to create a somewhat accurate image. CLIP also contains a text encoding model and an image encoding model. This is used to calculate how similar an image is to a text prompt.
The next model that is used to generate images is called diffusion. Just like CLIP, diffusion is very advanced. It works by taking an image and adding noise to it until it is no longer recognizable. Then it reconstructs the image, so the algorithm can learn how images are constructed. The diffusion and CLIP models do a very good job at creating high-quality images, but sometimes they mess up.
When I entered the prompt “silver PT Cruiser on a beach during the day, digital art,” the model struggled to get the shape of the PT Cruiser correct. However, it did a great job on the rest of the image. I believe that this is due to the lack of training data on PT Cruisers, along with the fact that they have a complex and unusual design. I came to this conclusion because when you use the same prompt but replace PT Cruiser with Jeep Wrangler, the AI almost perfectly replicates the shape of the Jeep. Jeep Wranglers are much more common cars with a simpler design. Therefore, there is probably more training data on Jeeps. It seems that the algorithms are only as good as the data that they are trained on.
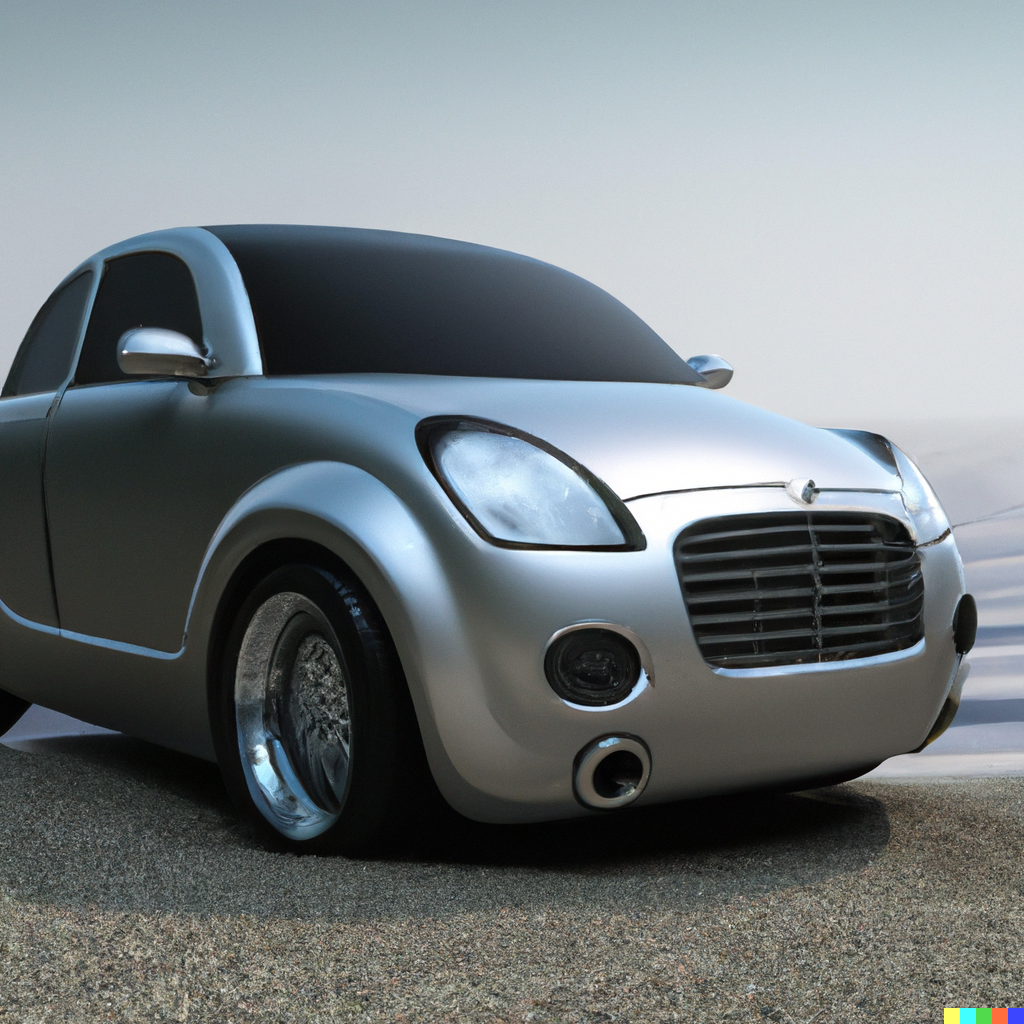
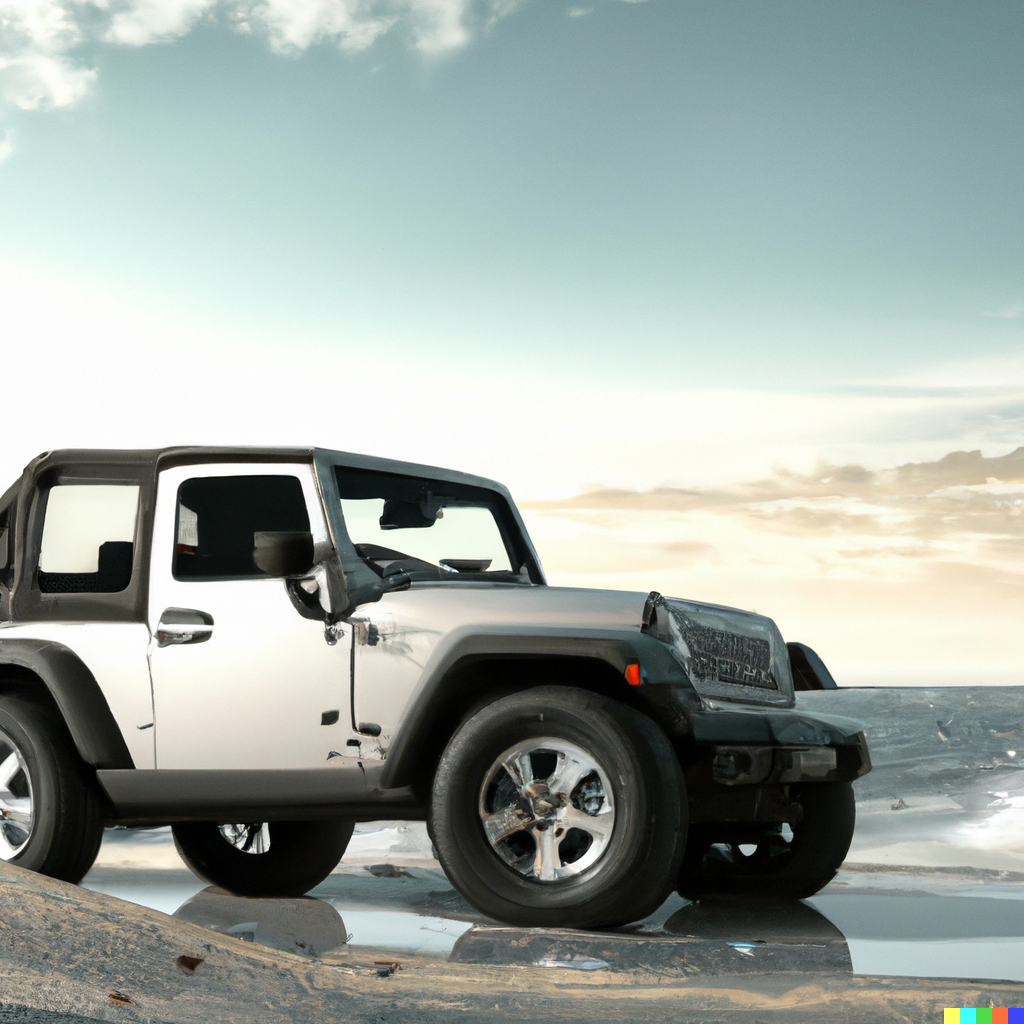
DALL-E 2 can generate extremely realistic images from any prompt user input thanks to these algorithms. However, like I said earlier, with great power comes great responsibility. The first issue with DALL-E 2 is the creation of biased images. We all know that the internet is not always the best place to get information. There are numerous false stereotypes floating around the internet. Through my research, I found that sometimes when you enter the prompt “Construction worker,” the AI generates all men. Another example is that if you use the prompt “CEO” again, it may generate all men. This aligns with stereotypes that are found throughout the internet.
Another issue with AI this powerful is how it can be used to create deep fakes of people. If you enter prompts containing celebrities, it will not work. But if you enter a form describing the physical properties of the celebrities, it may create an image that looks a lot like them. This is an issue because it can be used to create extremely realistic images that are not factual. Deepfakes are commonly used to spread fake news; therefore, the images generated by DALL-E 2 can be used for malicious purposes.
OpenAI has realized these issues, and they are actively working on solutions. So far, they have tried to edit the training data to dispel stereotypes. An example is that they could add images of woman CEOs or construction workers in the training data. Another solution is that they can change where they get their training data from. However, there are no other sources that provide as much information as the internet. Therefore, they should simply change which websites they scrape images from. This will allow DALL-E to create high-quality, unbiased images.
Talks of editing training data also have their own set of problems. Most artificial intelligence is only as smart as the data it is trained on. If someone can edit the training data, they can make the AI change its “beliefs” to those of the person who controls the data. We don’t need to worry about this too much with DALL-E 2 because it was made to showcase how far we have come with AI. But in the future, the centralization of AI training data may become an issue because whoever controls the data controls the AI.
In conclusion, DALL-E 2 has come a long way from the original DALL-E. Its ability to understand complex language and generate high-quality images has the potential to revolutionize how we get images. However, it is important to consider the potential challenges and risks that come with using such a powerful tool, such as bias and the possibility of misuse. As we continue to experiment with pushing the limits of AI, it is important that we do so with an eye towards responsible and safe use.